For a business to effectively make sense of this data from various sources, it needs to be data mapped, integrated, and standardized with a data dictionary.
To get a standardized view of structured data, businesses often need to use either ETL (Extract, Transform, and Load) or ELT (Extract, Load, and Transform) to make sure the schema, naming conventions, and formatting are consistent across all their different data sources to make analysis easier.
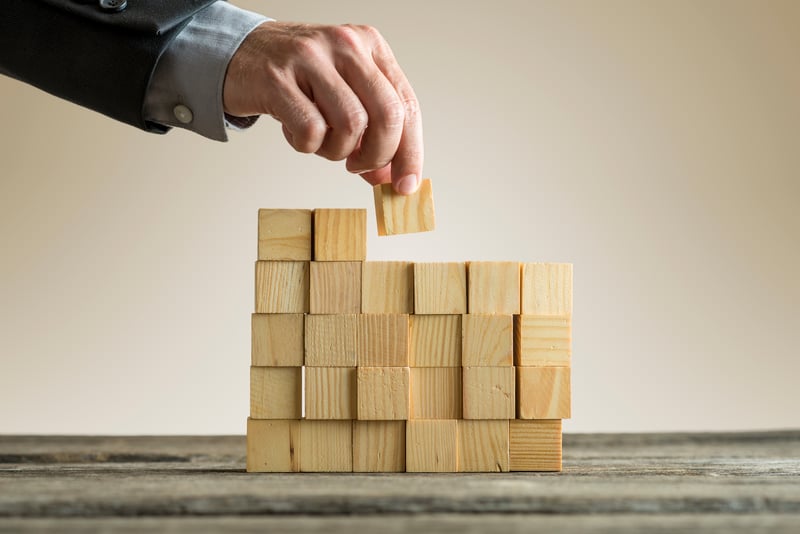
What is unstructured data?
Unstructured data tends to be qualitative in nature and doesn't follow a specific logical format or structure. This makes it more difficult to organize, measure, and analyze.
Some examples of unstructured data include:
- Emails
- Videos
- Audio
- Images
- PR coverage
- Documents
- Customer reviews
- Social media posts
If you imagine having a data lake that has thousands of videos, documents, and emails, with none of them having a consistent structure - it’s easy to understand how it might be difficult to extract meaningful insights.
This is why historically, a lot of unstructured data has just sat dormant in data lakes or cloud storage without being analyzed.
But by not analyzing their unstructured data, businesses are missing a lot of rich insight that can really help them understand trends and customer sentiments to improve business performance.
For example, analyzing customer reviews can provide valuable insights into product satisfaction and identify areas for improvement. Or the analysis of social media posts that mention a brand name can help a business understand customer sentiment toward the brand and uncover trends.
In the modern data-driven world, businesses that embrace data science, machine learning, and AI to gain insights from their unstructured data will give themselves a competitive advantage.
Key differences between structured and unstructured data
We’ve put together a useful table to help you understand the main differences in how structured and unstructured data is stored, processed, analyzed, and used across businesses:
Structured data |
Unstructured data |
|
Storage |
Typically stored in relational databases and data warehouses, in tables with predefined schemas. |
Unstructured data storage is usually in data lakes or file systems, where it’s stored in its raw state without a predefined schema. |
Processing |
Structured data can be processed and transformed using ETL or ELT tools, or by using SQL to standardize and join data to extract meaningful insights. |
Processing unstructured data often involves techniques like natural language processing (NLP), image recognition, AI, and machine learning. |
Analysis |
Structured data lends itself well to statistical analysis and trend forecasting. It’s easy to identify patterns and make decisions. |
Unstructured data analysis helps explore sentiments, opinions, and trends in customer reviews, social media posts, and other unstructured sources. |
Scalability |
Scaling structured data is often straightforward but can be costly as it requires more processing power from data warehouses or BI tools. |
It’s often more affordable to store large amounts of unstructured data in solutions like data lakes. |
Use Cases |
Structured data is commonly used in marketing performance analysis, financial reporting, inventory management, and customer relationship management. |
Unstructured data has applications in social media monitoring, customer sentiment analysis, and brand reputation tracking. |
Benefits of structured data for businesses
When your business has a single source of truth for your structured data, it can empower teams with the ability to easily analyze and compare the metrics that are important for their performance, and gain insights that help them make data-driven decisions. This is called data democratization.
The quantitative insights delivered by structured data can help decision-making at all levels of your business, from strategic planning by the C-suite to day-to-day paid search campaign adjustments by marketing managers.
Structured data can also be used in a wide range of business intelligence and data visualization tools, which helps transform your standardized data into interactive dashboards and custom reports. For example, your marketing team might use a tool like Tableau or Power BI to create a dashboard from structured data that track campaign performance metrics in real-time, enabling quick adjustments to strategies.
Structured data also opens up possibilities for automation within your business. For example, your marketing team might have a real-time data dashboard that calculates a blended ROI across all marketing channels. With accurate structured data, it’s possible to set up automation for bid or budget adjustments on particular marketing channels if the blended ROI drops below a particular level, helping to protect the profitability of your marketing activity.
Benefits of unstructured data for businesses
Unstructured data can often be a goldmine of qualitative insights that provides a more nuanced understanding of your customers, their needs, and their perceptions of your brand.
One of the key benefits of unstructured data is its ability to reveal hidden trends and sentiments. For example, by analyzing the content of customer reviews and social media posts, you can gain a real-time understanding of how your products or services are being perceived in the market.
Sentiment analysis tools can be used to automatically categorize these comments as positive or negative, and even identify common themes or issues. This can help your business respond quickly to customer concerns, improve your product or service offerings, and even manage your brand reputation more effectively.
Unstructured data can also help with product development and innovation. By analyzing customer feedback your business might identify new opportunities and areas for improvement that may not be apparent from structured data alone.
Another way that unstructured data can benefit is by helping you understand broader market trends and shifts in consumer behavior. For instance, by analyzing blog posts, news articles, and social media discussions in your industry, you’re able to identify emerging trends and potential threats.
Leveraging both structured and unstructured data: a holistic approach
For businesses that truly want to make effective data-driven decisions, it's not helpful to think of structured and unstructured data as mutually exclusive.
It shouldn’t be seen as structured Vs. unstructured data, but structured and unstructured data.
By leveraging both types of data, you’re able to make more informed, strategic decisions - with structured data providing a clear, quantifiable snapshot of your business performance, and unstructured data adding qualitative context to this picture.
However, to leverage both structured and unstructured data effectively, it’s important to invest in the correct tools.
Adverity is a market-leading integrated data platform that can connect all of your business's structured and unstructured data sources, and load both types of data to any data destination.
For example, Adverity can automate the process of extracting, cleaning, transforming, enriching, and loading your structured data from all of your marketing platforms and loading it into your data warehouse for analysis by your BI tool. At the same time, it can bring all customer feedback emails into a data lake for analysis by your data team.
Find out how Adore Me used the Adverity platform to finally understand their true global CPA across channels and improved their marketing strategies, or book a demo to discover how Adverity can help your business.