Data governance is a broad and complex topic involving the overall management of your business data's accuracy, availability, usability, integrity, and security.
Controlling the quality of your data is critical to effective data governance. Without the right data quality control measures in place, businesses run the risk of putting their trust in inaccurate or inconsistent data, which can lead to misguided decisions.
In this article, we’re going to take a deeper look at the importance of data quality control, recommend some practical measures for how to improve data quality, and introduce some ways in which Adverity can help solve data quality issues.
The importance of data quality control
Having high-quality data you can rely on helps you foster a data-driven culture within your organization. This leads to better collaboration, decision-making, and business performance - enabling your teams to optimize more effectively, identify new opportunities, and stay ahead of the competition.
On the other hand, poor quality or erroneous data can lead to a lack of trust in business information, resulting in reduced collaboration, missed opportunities, inaccurate campaign optimizations, and a detrimental impact on overall business performance.
Working with a data integration solution is one way to help improve the quality of your data. But data integration alone is not enough - you also need the right data quality control measures.
From one perspective, having no data integration process can actually be less risky than having data integration without quality control.
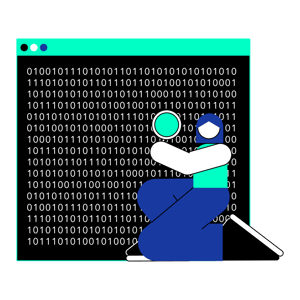
Imagine a marketing team operating without data integration from a dozen different marketing sources. They have to make optimization decisions from the data on each individual platform. It’s time-consuming. It’s inefficient. It’s not the most accurate way to compare cross-channel performance. But at least the marketing team is aware of the challenges and limitations.
Now, imagine the data team putting together a data integration solution to bring all these different marketing sources together - but without the right data quality control measures.
The marketing team is excited about this single source of truth, being able to compare channels more accurately, and also about the amount of time they will be able to save by not having to log into multiple different platforms. They trust that the data is going to be complete, accurate, and timely.
But it isn’t.
Inconsistencies in date formats and errors in data fetches mean that the data for some channels isn’t correct. But this doesn’t get identified. The marketing team, with a false sense of security, assumes that everything is okay and makes optimization decisions based on inaccurate data. They move the budget from one of their best-performing campaigns that isn’t being reported correctly to a less effective campaign simply because quality control measures weren’t in place.
That’s why having the right data quality control measures in place is so important. If errors make their way into your centralized database without measures to pick them up, users across the business could be using erroneous data without anyone noticing.
As part of your data governance strategy, data quality control addresses this by monitoring and detecting errors within your business data so corrective action can be taken.
The management of data quality control
When considering how to approach data quality management, it’s important to understand the five primary dimensions of data quality: accuracy, completeness, consistency, timeliness, and relevance.
If you’re integrating your data into a centralized, standardized database - you’re going to need a robust data quality control process that’s focused on the measurement and management of each of these five dimensions.
Accuracy
For data to be accurate, it needs to be free from any errors.
Monitoring this manually as part of a data quality control process will require regular spot checks between consolidated data and the original data sources. This can be a time-consuming process, and it’s simply not feasible to manually check all available data.
Forunately, this is a process that you can automate with the help of anomaly detection, which alerts data owners when any consolidated values appear outside of the expected range, enabling you to look into the issue immediately and correct any errors.
Completeness
For your data to satisfy the completeness dimension of data quality, it’s imperative that all relevant data is present and no part of it is missing.
This can be incredibly difficult to monitor without the right tools and technology.
To help automate this task as part of your data quality control process, Adverity has an advanced Activity Monitor that tracks all data integration tasks, from extraction to transformation, to transfer into your chosen data destination.
This gives your business visibility of all your data integration tasks and ensures that all your data has been fully uploaded.
Consistency
High-quality data must also be consistent in terms of naming conventions, formatting, and structure.
This aspect of data quality management is something that many businesses find challenging when consolidating data due to the different naming of fields and the different formats that platforms use for things like date values, performance metrics, and currencies.
Data integration platforms typically have a range of automation features that can help ensure that your consolidated data is consistent and avoid data quality issues.
At a basic level, data mapping can be applied to each one of your data streams to ensure that the correct values are passed through to the right unified data fields in your centralized database.
Data transformation and enrichment features can help ensure the consistent formatting of your data, with currency conversion, location unification, and language translation available as out-of-the-box enrichments with Adverity
Some data integration platforms also have advanced functionality like Smart Naming Conventions, which helps ensure that values within your database adhere to specific conventions and either alerts you or prevents data upload if any non-compliant values are found.
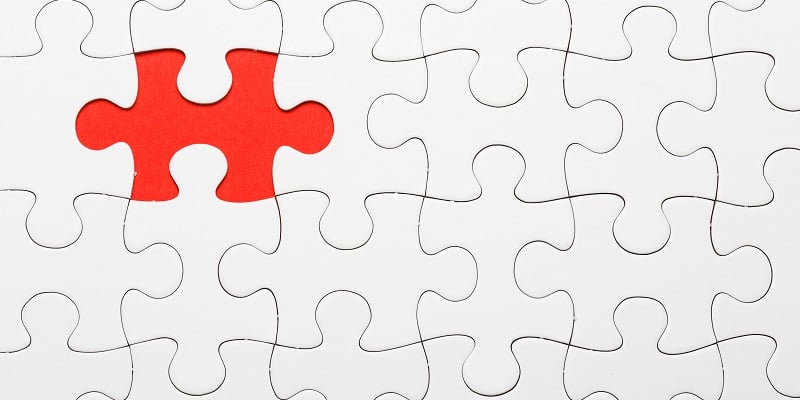
Timeliness
To meet data quality standards, your data should be up-to-date and available when needed.
Not only does Adverity have a market-leading data fetch frequency (up to every 15 minutes), but the Performance Manager helps provide in-depth insight into the performance of your data sources, breaking it down into the time it took to fetch, transform, and load.
By becoming aware of any data streams that are taking longer to integrate than might be expected, you can proactively address any issues to improve the timeliness of your data.
Relevance
Relevance is more of a qualitative measure than the other four dimensions. As such, it’s harder to put robust data quality controls around.
However, as a best practice tip, consider regularly checking in with the marketing teams to ensure that your consolidated data is still fit for purpose and that they are getting everything they need in your centralized database.
You might also query if your marketing team is receiving any data they no longer need.
By choosing the right data integration platform, you’ll have complete control over the data brought into your database, making it quick and easy to modify the information consolidated from each data source.
Best practices to improve data quality control
Now that you understand the importance of data quality control, it’s time to look at some of the data quality best practices you can put into practice to achieve it.
Implement a data governance strategy
Data governance sets the foundation for maintaining data quality control by establishing guidelines, frameworks, and standards for managing your business data and ensuring its accuracy.
It's important to choose a data integration platform that offers a wide variety of data governance features to help your business implement an effective strategy that aligns with the seven building blocks of good data governance.
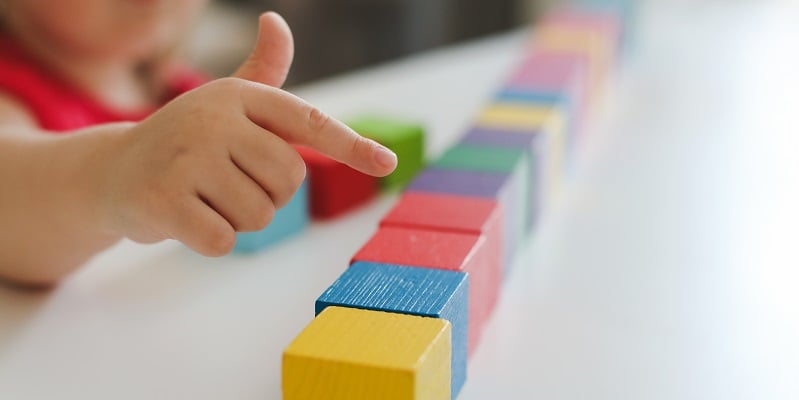
By choosing the right platform, you can automate the data integration process, reducing the risk of human error and improving the quality of your data.
Implement the right authorization controls
To implement robust data quality control, you need to set permissions around who can make adjustments to each of your different data streams.
By putting controls around this, you can maintain the integrity of your data and prevent any unauthorized changes, so you can have peace of mind that your integrations meet data quality standards.
That's why it's essential to select a data integration solution that is capable of storing all the various credentials authorized to connect to and modify each of your data streams.
Standardize and validate your data
To ensure your data is suitable for effective comparison and analysis, you must ensure it is standardized and validated.
This can be a challenging process to complete manually,
By using a data integration platform, you can automate the standardization and validation of data - with the functionality available to alert stakeholders if values don’t meet agreed criteria.
Use the right monitoring tools
Keeping on top of data quality control can be tough, especially for larger businesses with vast amounts of data from multiple sources.
In many cases, it’s simply not feasible without the support of the right tools.
Data integration platforms can help take a lot of the manual work out of data quality control monitoring by immediately identifying any data that sits outside the expected range. Tools like Adverity’s Performance Manager help data teams track the timeliness and completeness of data transfers.
Complete regular spot checks on data
Automated data integration platforms allow you to improve the speed, accuracy, and quality of your data consolidation, with many of the leading platforms also having specific functionality to help with the data quality control process.
However, technology doesn’t have the context to understand the significance or the "why" behind certain data patterns, trends, or anomalies. For example, a steep increase in conversion rate around a time-limited promotion might be correctly flagged as an anomaly by an algorithm (and it would be right!), but human intervention would help explain why - and that it’s correct and not an error.
That’s why having human eyes on your data is important.
As part of a robust data governance strategy, it’s important to schedule regular manual checks of consolidated data to help identify and rationalize any discrepancies or anomalies, ensuring that it remains accurate and complete.
Vodafone: Improving data quality with Adverity
As one of the world’s leading telecommunications providers, it will come as no surprise that Vodafone Germany has an incredibly mature online marketing strategy involving 20 different channels and more than 150 marketing campaigns annually.
However, although their marketing performance was strong and successful, their marketing data management and analysis led to frustrations and inefficiencies across the business.
Much of their data integration was being completed manually, and some sources weren’t easy to integrate - leading to data silos, a lack of visibility and collaboration across the team, and no consistent data quality control measures.
Vodafone decided it was time to act and chose Adverity as their data integration platform for “Project Neuron” - a Neutral, User-friendly, Real-time measurement Online Platform to evaluate and analyze marketing activity.
Adverity enabled the real-time integration of Vodafone’s 20 marketing sources into a centralized data lake, eliminating the data silos and providing the marketing team with a single source of truth, fully automating error detection and identification of anomalies.
Project Neuron was a huge success, with a 20% reduction in time spent on data acquisition and an increase of 80% in data quality. For more on this, you can read the full case study here.
Elevate your data quality control processes with Adverity
Ensuring data quality control is an important part of any effective data governance strategy.
High-quality data fosters a data-driven culture, promotes better decision-making, and strengthens business performance.
Staying on top of data quality control manually can be challenging for businesses managing large amounts of data.
For many businesses, implementing the right tools and technology to make the process manageable is the only sensible solution.
As a leading data integration platform, Adverity has a broad range of features and functionality that can help with data quality control as part of your broader data governance strategy.