What is data quality?
An In-Depth Guide for Marketers to Achieving High Standards
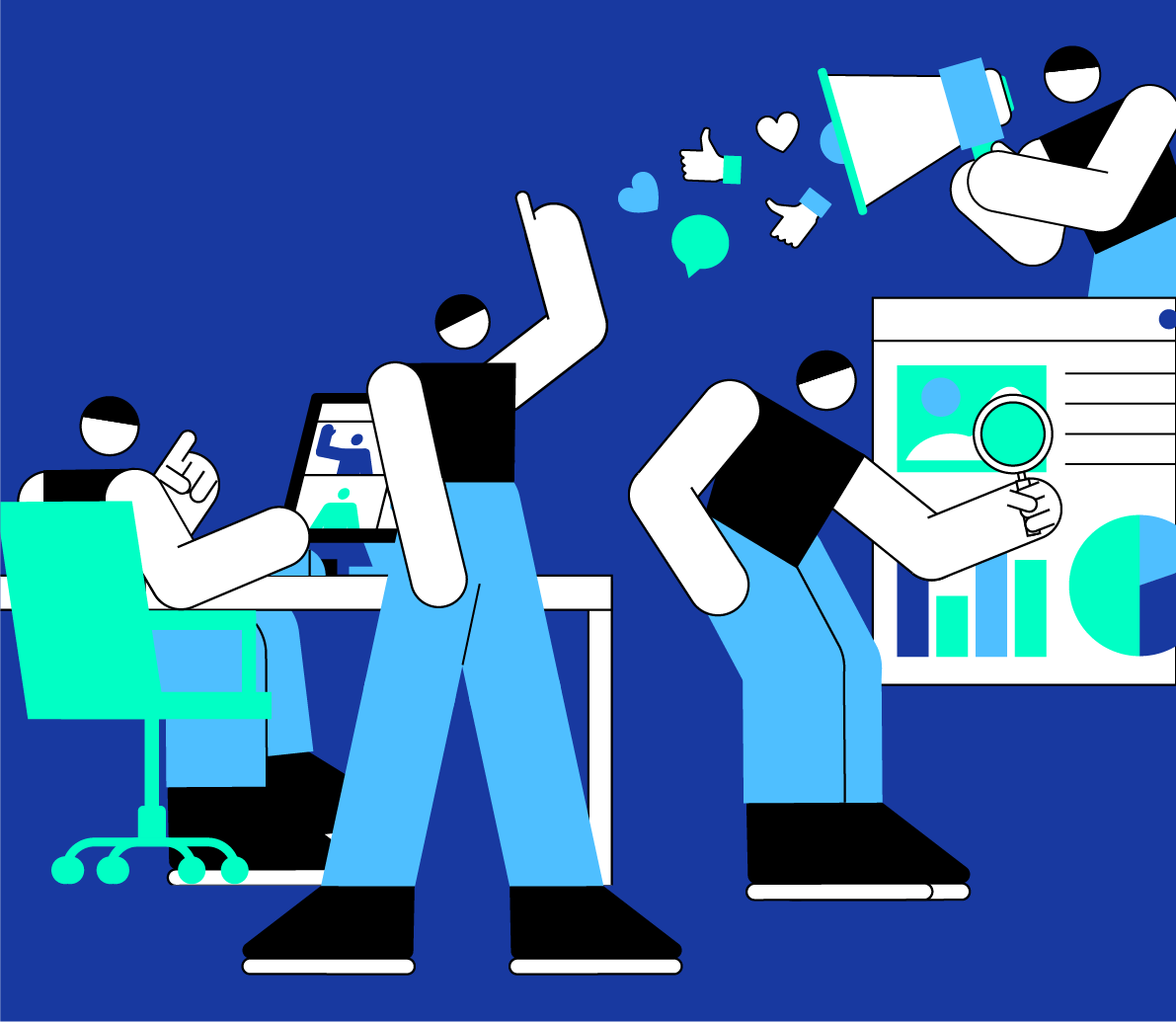
For modern marketers, data forms the foundation of every decision, from targeting the right audience to allocating budget for channels. But what happens when the quality of your data dips?
Inevitably, the cracks start to show.
Inaccurate or incomplete data leads to misleading insights, poor decisions, and, ultimately, wasted resources. In fact, Gartner estimates that poor data quality costs organizations an average of $12.9 million annually.
This guide explores the six key dimensions of data quality, providing marketers with a framework to build a solid data foundation. Good data quality leads to better decisions, and better decisions drive higher ROI.
It’s that simple: data quality is the essential foundation for any meaningful analysis.
Data quality refers to the overall health and reliability of your marketing data. Think of it as a fitness check for your data assets: are they accurate, complete, consistent, unique, timely, and valid? When your data meets these standards, it becomes a trusted foundation for making informed decisions, running effective campaigns, and uncovering key insights.
On the other hand, poor-quality data can lead to costly mistakes—like sending promotions to outdated contacts or making critical strategic choices based on flawed information. These errors can damage customer trust, inflate budgets, and undermine the performance of your marketing efforts.
Simply put, data quality is the gold standard for ensuring that your data is fit for purpose. It’s what guarantees that your marketing data is reliable enough to act on with confidence.
Data quality dimensions are measurable characteristics that help assess whether data is fit for its intended use.
Here's a quick overview:
These dimensions offer a structured approach to evaluating data’s integrity, ensuring that it can be trusted for decision-making. They’re the building blocks of good data quality, and absolutely essential for modern marketers.
So, with that in mind, here’s a more in-depth look at what the data quality dimensions are, why they matter, and how to get them right.
Data accuracy refers to how closely data reflects real-world events, ensuring it matches reality without errors or distortions. Data that closely reflects the real-world events it represents is accurate.
Accurate data provides a true representation of metrics such as customer behavior, sales performance, and campaign outcomes. When data is inaccurate, marketers might target the wrong audience, allocate budgets inefficiently, or draw incorrect conclusions about campaign performance. This can result in lost revenue, decreased customer trust, and reduced marketing ROI. Accuracy ensures that marketers have a reliable foundation for analysis and decision-making.
To maintain data accuracy, marketers should implement regular data validation and cleansing practices. This involves checking for discrepancies, removing errors, and cross-referencing data with trusted sources. Tools like automated data cleansing software can help maintain a high standard of accuracy by flagging anomalies.
Completeness measures whether all necessary data is present.
Complete data gives a full picture of marketing performance. Incomplete data can cause marketers to miss crucial insights that would help in better targeting and segmentation, ultimately weakening campaign outcomes and eroding trust in data quality. When missing data goes unnoticed, it can distort results, leading to bad decisions, wasted resources, and ineffective strategies.
Completeness can be ensured through the implementation of validation checks at data entry points. Marketers should also use data integration tools that flag missing fields, enabling quick corrections. Regular audits of the dataset can also highlight gaps that need to be filled.
Data consistency ensures that data remains uniformly formatted and synchronized across different systems and platforms.
Consistency is crucial for ensuring that marketers can rely on data no matter which system they’re using. When data is inconsistent, it can cause confusion, errors in reporting, and conflicting insights across platforms, making it difficult for marketers to trust their data and draw reliable conclusions.
Achieving consistency requires implementing data governance policies that standardize formats, terminologies, and units across all platforms. Using data integration tools that automate this standardization can greatly help in ensuring consistent data across your systems.
Duplicate data inflates metrics and leads to inaccurate assessments of marketing performance. For instance, duplicate customer records can result in multiple communications to the same person, damaging customer relationships. Additionally, it increases operational costs as redundant data takes up unnecessary storage space, leading to inefficiencies in overall marketing strategies.
Using data deduplication tools can help automatically detect and remove duplicate entries. Marketers should also enforce strict data entry rules to minimize the chances of duplicates entering the system in the first place.
In marketing, where customer preferences and trends shift quickly, having up-to-date data is essential for timely decision-making. Timely data allows marketers to respond quickly to changes, optimize campaigns in real-time, and improve targeting. On the flip side, using outdated data can result in missed opportunities and wasted spend by targeting customers with irrelevant offers or basing strategies on insights that are no longer relevant.
Implementing automated data pipelines ensures that data is constantly updated and relevant. Marketers should also establish schedules for regular data updates and set policies that determine acceptable timeframes for when data should be refreshed.
Validity refers to whether data conforms to the required format, structure, or rules. Data that adheres to predefined business rules, such as correct date formats or mandatory fields, is considered valid.
Validity ensures that data can be reliably used without additional manipulation or correction. Invalid data can lead to operational inefficiencies and errors that reduce the effectiveness of campaigns. For example, if a dataset contains invalid email addresses, it can result in failed communication efforts, affecting the efficiency of marketing campaigns.
Marketers can maintain data validity by setting up validation rules within their data entry systems. These rules should enforce consistency in formats, required fields, and other predefined standards. Regular data audits can help identify and correct invalid data entries.
Ensuring high data quality involves more than just addressing one or two dimensions. Marketers must tackle all six dimensions—accuracy, completeness, consistency, uniqueness, timeliness, and validity—to create a reliable foundation for their campaigns.
By focusing on each aspect, marketing teams can optimize their strategies, improve targeting, and ultimately boost their return on investment.