Marketing Mix Modeling is often touted as a potential solution to the increasing difficulty of multi-touch attribution. But what is it and how does it work?
With cookie deprecation spelling the end of multi-touch attribution, Market Mix Modeling (MMM) is often seen as a critical solution for many businesses to maintain a holistic view of channel performance.
In this blog, we explore what market mix modeling is, how it works, and why it is a useful tool for marketers amid the loss of third-party cookies.
What is Marketing Mix Modeling?
Marketing Mix Modeling (sometimes called Market Mix Modeling or Media Mix Modeling) is a method for measuring the performance of different marketing channels using aggregated data.
While methods such as attribution modeling take a bottom-up approach, analyzing how different channels and campaigns contribute to sales at an individual user level, MMM uses statistical modeling to find correlations between marketing investments and returns at a macro level.
How does Marketing Mix Modeling work?
MMM collects aggregated data from a wide range of both marketing and non-marketing sources, over a given period (normally several years). This data is then used to determine how much each factor historically impacted your sales and then uses this to calculate the likely impact of future campaigns on any given channel with any given budget.
An example of Marketing Mix Modeling
Ok, so over a particular period of time let's say you run a Google Ads campaign, then you run a Facebook Ad campaign, and then you run Google and Facebook Ads campaigns at the same time.
Now during this period, your sales are going up and down and, by comparing how revenue changed, you can start to build a picture of what the likely impact of each campaign was.
For example, if during your Google Ads campaign sales increased by X amount and during our Facebook Ads campaign sales increased by Y amount, you can get a good idea of how much each contributed to sales when you ran both campaigns at the same time.
More importantly, you can use that information when planning future campaigns to deliver more sales and optimize the returns you're getting from your marketing spend.
Is it really that simple?
No, not really. In fact not at all. In reality, it is much more complex than that. Firstly, there are a lot of variables that need to be incorporated into an MMM calculation. A lot of variables. Things like promotions, market conditions, competitor activity - even seasonality. Basically, anything that might affect user behavior needs to be taken into account.
Plus, you will most likely be running multiple marketing campaigns across multiple channels which all need to be tracked. And, for all the campaigns you are running, your marketing spend is going up and down during those campaigns - and all this needs to be factored in.
Secondly, you're going to have to get a lot more data than just a few months’ worth. In fact, for most standard MMM models to give accurate results, you are going to need at least two years of historical data.
Why is Marketing Mix Modeling useful for Marketers?
Bit of an obvious one but the main benefit of MMM is that it allows marketers to get a holistic view of channel performance and make adjustments to spend in order to optimize each campaign and maximize the ROI. At the same time, it can help marketers to understand how different campaigns are adding incremental value to sales.
Perhaps more crucially, as cookie deprecation reduces the scope of multi-touch attribution, MMM does not rely on third-party cookies and therefore has enormous value for marketing teams that still want to be able to attribute revenue to different streams. So much so, that it is often touted as one of the key solutions to cookie deprecation.
What are the limitations of Marketing Mix Modeling?
The biggest limitations associated with MMM are the time and resources the method demands. Because of the complexity, traditionally, MMM calculations take a long time to update with new data. What this means is that, when you start updating your model with all the data from the previous 3 months at the end of Q1, you still need to wait another 4 to 6 weeks before the results are ready, at which point they may or may not be relevant anymore and you’re already halfway through Q2 anyway.
Naturally, in the rapidly moving digital online world we live in, this time to value is far from ideal. That's why today, most businesses utilize the power of more advanced AI analytics platforms to deliver what is known as Continuous MMM.
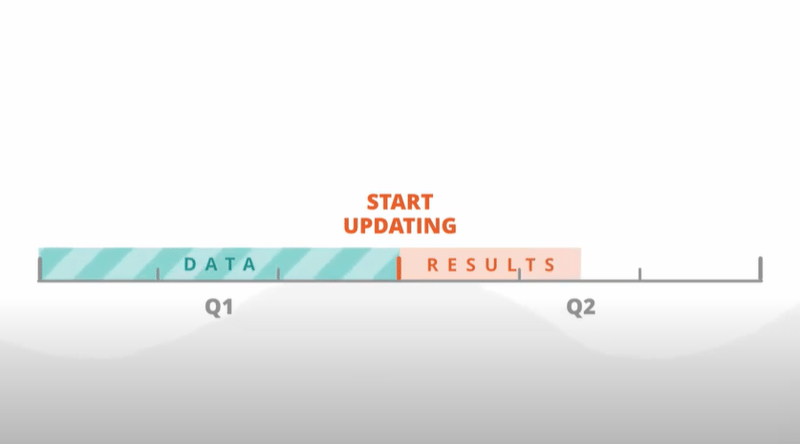
What is Continuous Marketing Mix Modeling?
As the name implies, Continuous MMM goes beyond the traditional project-based approach to MMM to provide continuous calculations. This means that marketers can make regular adjustments to channel spend based on real-time data into performance.
Because continuous MMM involves a tremendous amount of calculations, it is generally impossible to do this manually. Instead, most businesses utilizing a continuous MMM approach rely on sophisticated tools and artificial intelligence.